Edgar Engel
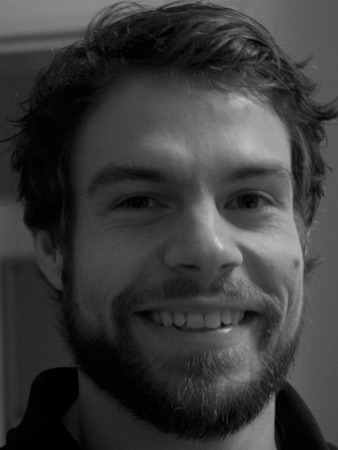
Dr Edgar Engel
Research Fellow at Trinity College
Fellow of Trinity College
Office: 514 Mott Bld
Phone: +44(0)1223 3 37278
Email: eae32 @ cam.ac.uk
TCM Group, Cavendish Laboratory
19 JJ Thomson Avenue,
Cambridge, CB3 0HE UK.

Research
I am a junior research fellow at Trinity College, Cambridge, working in the Theory of Condensed Matter Group, University of Cambridge. My research focusses on leveraging machine-learning techniques to enable and accelerate the prediction and design of complex materials and their properties from first-principles.
Towards computational materials design from first-principles
One of the aspirations of the computational sciences is to accelerate the discovery of novel, technologically-interesting materials and, ideally, to design revolutionary functional materials from first-principles., The canonical workflow for the computational design of (crystalline) materials involves searching the space of computationally stable structures for candidates with desirable properties, followed by predictive assessment of their phase behaviour to distil those, for which it is worthwhile to attempt experimental realisation.
My research focusses on leveraging machine-learning (ML) techniques to address key bottlenecks that arise in the context of complex, flexible materials, such as bio-molecular crystals. Molecular crystals are not only ubiquitous in the pharmaceutical industry but also show great promise for applications ranging from photovoltaics to gas absorption. Their tendency to exhibit structural polymorphism provides a mechanism for tuning properties by controlling structure, but also introduces the challenge of stabilising polymorphs with desired properties.
Rationalising and screening structure data
To date the capacity of crystal structure prediction (CSP) for discovering materials at a rate that surpasses experiment by orders of magnitude relies on many ad hoc approximations. These permit sidestepping otherwise preventative computational costs, but compromise reliability, in particular for complex materials. Hence the appeal of means of screening structure data. My interest lies in the use of unsupervised, semi-supervised, and supervised ML schemes for rationalising structure data based on measures of structural similarity. These provide universal, data-driven means of elucidating structure-property relations, removing redundant structures, and extracting minimal sets of representatives for subsequent rigorous investigation. Structure data might also come from high-throughput crystallisation experiments, where conclusive studies of the properties and phase behaviour of structures are often prevented by limited sample quantities.
First-principles thermodynamics
Understanding thermodynamic stability is sufficient (although not necessary) to ensure long-term stability. However, the often minuscule free energy differences between polymorphs of molecular crystals pose a formidable challenge, and the success of CSP is often limited by the inability to routinely predict the relative stability of competing polymorphs. My interest is in leveraging ML approaches to enable rigorous first-principles, path-integral free energy calculations, which are otherwise not computationally viable. Such free energy calculations permit predicting full p−T phase diagrams, and provide a comprehensive understanding of the importance and interplay of subtle physical effects, such as the electronic structure, the statistical mechanics of the nuclei and the simulation cell, and thermal expansion. They naturally generalise to other classes of complex materials, and, where required, to the use of highly-accurate quantum-chemical electronic structure methods.
Complementing experimental structure determinations
The final step in a CSP study is the experimental verification of a proposed structure and its properties. Nuclear magnetic resonance (NMR) spectroscopy is particularly well suited to determining the structure of (bio-)molecular crystals, which generally contain hydrogen atoms and often come in small sample quantity and/or powdered form. Structure determination usually proceeds by comparing experimentally observed NMR chemical shifts with those predicted for candidate structures. Its reliability depends on the uncertainties in the predicted shifts, arising from the descriptions of the electronic structure and the statistical mechanics of the nuclei and simulation cell. Minimising these uncertainties optimises the resolving power of NMR spectroscopy. Chemical shifts are traditionally computed by first-principles electronic-structure methods. However, their computational costs limit the complexity of materials they can be applied to, and effectively prevent shift predictions accounting for quantum and thermal fluctuations of the nuclei and simulation cell. ML techniques provide an avenue for predictive calculations of finite-temperature chemical shifts for large, flexible, complex systems, such as bio-molecular crystals, and promise to improve the sensitivity of NMR crystallography.
Properties predictions at finite temperature
The importance of the statistical mechanics of the nuclei (and simulation cell in periodic systems) is not limited to NMR chemical shifts but extends to many materials properties, electronic and mechanical, in particular for soft, flexible materials. For instance, the electronic and optical gaps of organic molecular crystals are of great interest for applications in photovoltaics.

In Plain English
Designing new functional materials on a computer and then realising them in a laboratory is a grand challenge -- for no class of materials more so than those composed of flexible, loosely-bound bio-molecules. These are proving a particularly hard nut to crack, because the number of similar, yet subtly different ways of stacking the molecular building blocks into ordered structures is vast, because their stability depends sensitively on a delicate balance of physical effects and conditions such as temperature and pressure, and because their experimental realisation and analysis is often complicated and costly.
My research focusses on the use of machine-learning strategies to structure the space of molecular materials, to identify relationships between materials properties and their microscopic structure, and to facilitate the otherwise preventively expensive calculations required to accurately predict their stability and properties (and their reaction to changes in temperature and pressure).
Featured Publications
- Liquid water contains the building blocks of diverse ice phases. Nature Comm. 11 5757 (2020)
- A Bayesian approach to NMR crystal structure determination. Phys. Chem. Chem. Phys. 21 23385 - 23400 (2019)
- Assessment of Approximate Methods for Anharmonic Free Energies J. Chem. Theory Comput. 15 5845 - 5857 (2019)
- Ab initio thermodynamics of liquid and solid water. P. Natl Acad. Sci. USA 116 1110 - 1115 (2019)
- Mapping uncharted territory in ice from zeolite networks to ice structures. Nature Comm. 9 2173 (2018)
- Anharmonic nuclear motion and the relative stability of hexagonal and cubic ice Phys. Rev. X 5 021033 (2015)